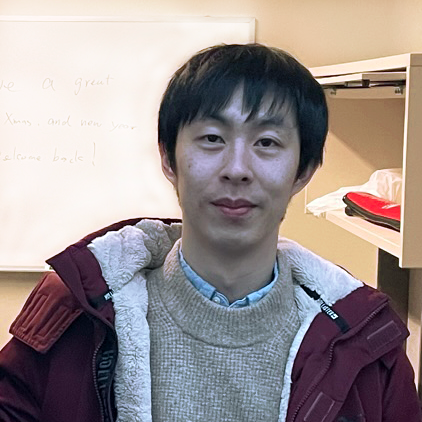
Post-Graduate Stories
Chi-Kuang Yeh Explores New Modelling Approaches for Early Diagnosis of Alzheimer’s Disease
Chi-Kuang Yeh’s research project, titled “Semi-supervised Learning for High-dimensional Functional Data Integration with Measurement Error” will explore new modelling approaches to deal with large-scale high-dimensional and functional data collected with different devices (and often contaminated with measurement error). He will apply the proposed methodologies to identify key imaging and genetic biomarkers for the early diagnosis of Alzheimer’s disease.
Program: CANSSI Distinguished Postdoctoral Fellowship
Region: National
Date: 2023–2025
Project Focus Areas
In the Alzheimer’s Disease (AD) Neuroimaging Initiative (ADNI) study, imaging, clinical, genetic and demographic datasets of patients are collected. However, because the data collection procedures are performed for different groups, when we attempt to integrate data from multiple sources, the differences in data quality can be a potential confounder and cause interested estimators to be biased.
The major goals of the proposed research are to develop powerful and innovative statistical learning methods and computational tools that can systematically handle different measurement error and misclassification mechanisms brought by the multisource/multimodality datasets. The project will address methodological and computational challenges in data integration including high-dimensionality, functional covariates, measurement error and statistical efficiency.
The project team is particularly interested in identifying key imaging and genetic biomarkers for the early diagnosis of AD where imaging data are of different qualities for some subjects.
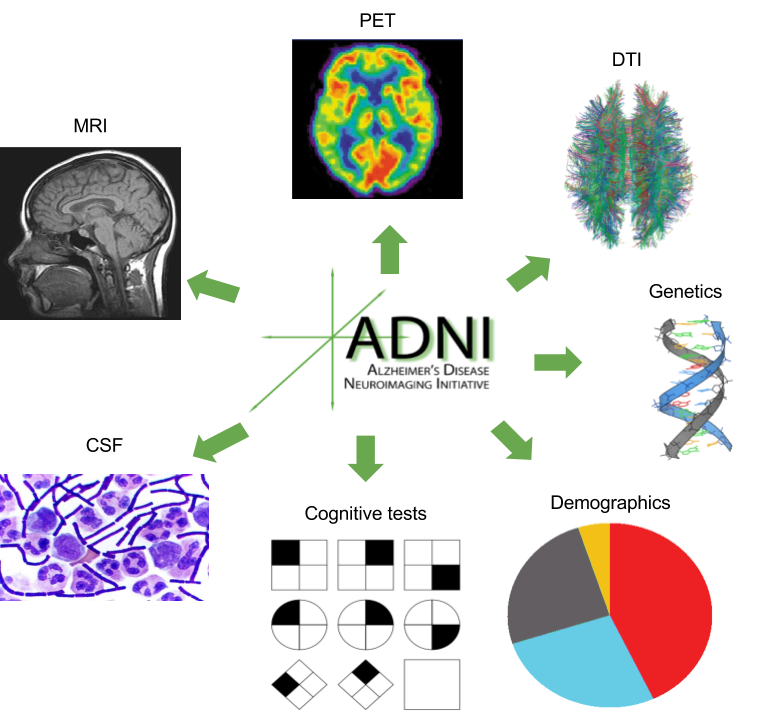
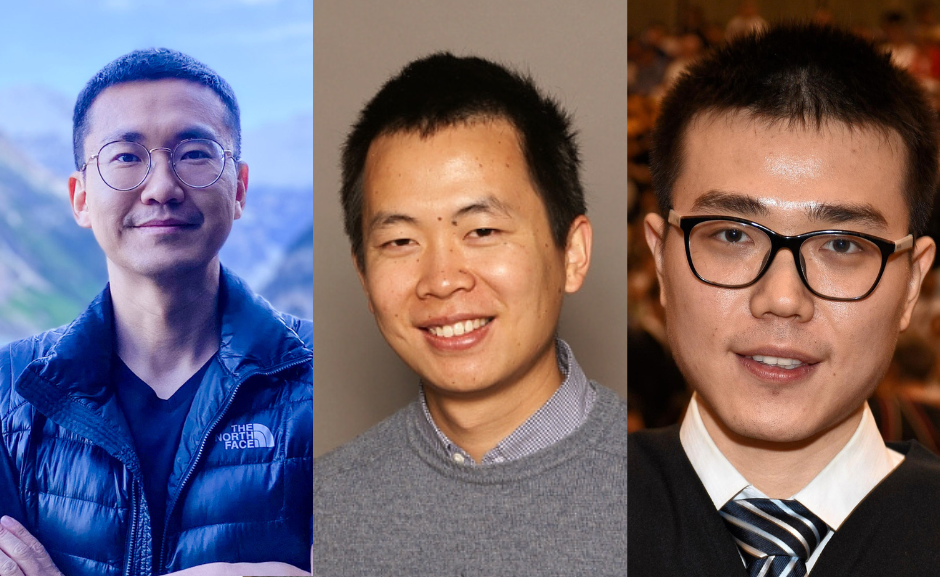
Getting to Know Chi-Kuang
Chi-Kuang Yeh received his PhD in statistics from the University of Waterloo in 2023.
His research program operates mainly in two directions: optimal design (OD) and functional data analysis (FDA). For OD, his focus is on proposing flexible and fast computational algorithms to numerically solve OD problems, with particular emphasis on the robustness of the results and applications for drug studies. As for FDA, he has developed new statistical methods on densely observed curves. The effectiveness of these methods was demonstrated in applications to model selection for daily electricity price curves.
Chi-Kuang has more than a decade of experience as a teaching assistant and notes that he has always had a keen interest in teaching, as it provides an opportunity to positively impact others in a common discipline. In pursuing his goal of an academic career, he aims to emphasize research and teaching equally.
As a recipient of the CANSSI Distinguished Postdoctoral Fellowship, Chi-Kuang will work under the supervision of Archer Yi Yang and Qihuang Zhang at McGill University and Peijun Sang at the University of Waterloo.
I have always been passionate about solving problems from real-life corners by integrating methods from different disciplines and delivering the findings to others.
About the Supervisors
Archer Yi Yang
Archer Yi Yang is an Associate Professor in the Department of Mathematics and Statistics at McGill University in Montreal.
He is also an Associate Academic Member of Mila (Quebec AI Institute), an Associate Member of the School of Computer Science at McGill, and a Member of the Quantitative Life Sciences program at McGill.
His research interests include the following areas:
- Statistical machine learning
- Statistical computing
- High-dimensional statistics
- Applied problems in genomics, medicine and industry
Peijun Sang
Peijun Sang joined the Department of Statistics and Actuarial Science at the University of Waterloo in September 2018 as an assistant professor.
His current research interest is focused on functional data analysis, which concerns curves and function-valued variables. Brain imaging data such as EEG signals, function magnetic resonance imaging (FMRI) and diffusion tensor imaging (DTI) are typical examples. He has a keen interest in applying functional data analysis techniques to study functional connectivity between these imaging data collected from different regions of the brain. Knowing these connectivities is of vital importance in practice, since it may provide new insights into some diseases that can hardly be diagnosed with traditional methods. In theoretical aspects, he is concerned about large sample properties of high dimensional functional regression models proposed for these data. Dependence modelling with copula models for discrete and/or survival outcomes also attracts his attention.
Qihuang Zhang
Qihuang Zhang is an assistant professor at McGill University, Department of Epidemiology, Biostatistics and Occupational Health. Prior to that, he was a post-doc working with Dr. Mingyao Li and Dr. Rui Xiao at the University of Pennsylvania. He received his Ph.D. from the Department of Statistics & Actuarial Science at the University of Waterloo (2017–2020), under the supervision of Dr. Grace Y. Yi. He was also a sessional lecturer at Western University.
His research centres around the development of statistical methods for genetic association studies, RNA sequencing, and spatial multi-omics. Basically, he strives to make sense of complex data by addressing measurement error, misclassification, graphical association structure, and the curse of dimensionality. Lately, he has been diving into the field of deep learning and working on developing methods to analyze spatial transcriptomics and spatial metabolomics data. These methods aim to provide valuable insights into the spatial organization of molecules, cells, and tissues from a statistician’s perspective, with the potential to yield significant benefits for medical research.
Explore More Stories
Find Related Programs
“Semi-supervised Learning for High-dimensional Functional Data Integration with Measurement Error” is a CANSSI Distinguished Postdoctoral Fellowship (CDPF) project. The CDPF is a two-year program that includes a substantial research project, applied interdisciplinary collaboration, and teaching experience.
CANSSI Distinguished Postdoctoral Fellowships are supported by a competitive salary. They provide opportunities for professional development and prepare postdoctoral fellows for success in a variety of careers.