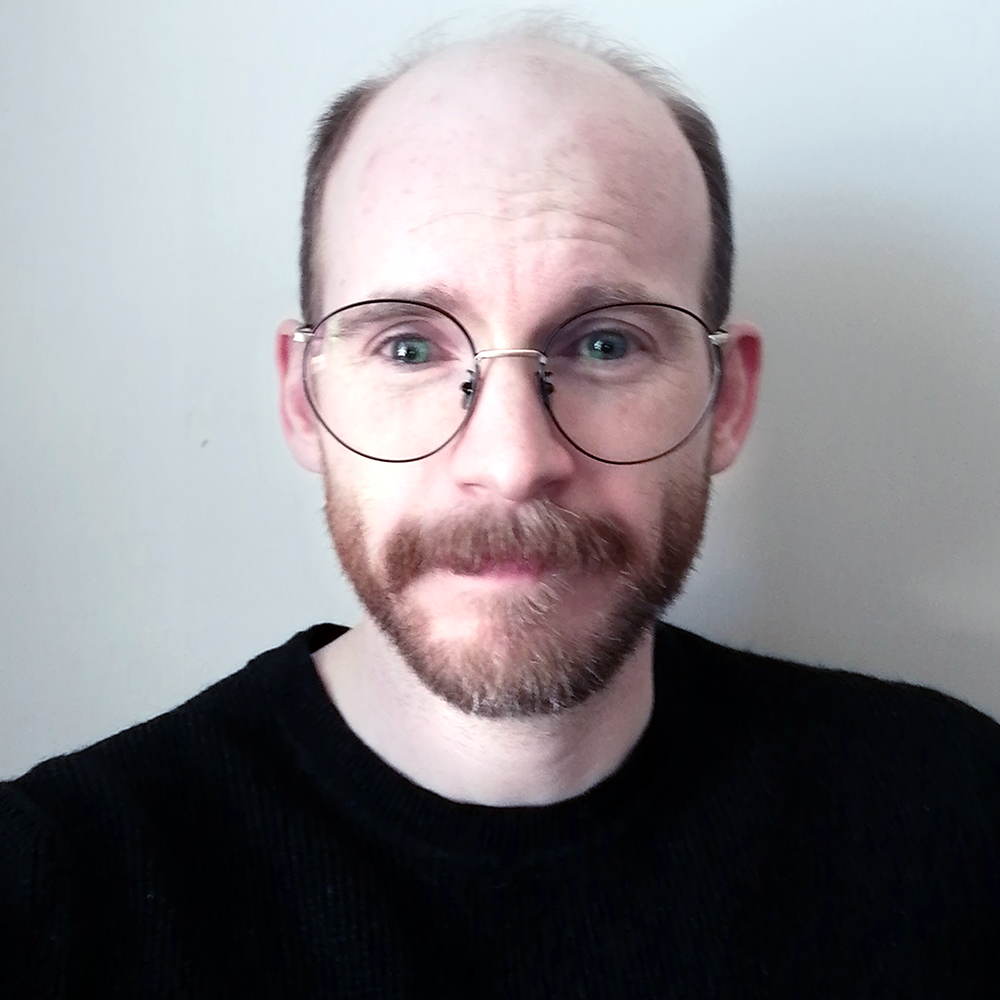
Post-Graduate Stories
Alex Sharp Explores “Statistical Foundations of Invariant Representation Learning, with Applications to Data-driven Compression and Algorithm Design”
Alex will develop novel deep learning methods based on notions of invariance and/or sufficiency for data compression and data-driven algorithm design (e.g., discrete optimization) and will develop specialized statistical theory for those settings.
Program: CANSSI Distinguished Postdoctoral Fellowship
Region: National
Date: 2023–2025
Project Focus Areas
Advances in deep learning methods have been driven largely by computation and engineering. Some of the most successful methods, however, incorporate notions of invariance and/or sufficiency. These properties are especially important for robustness to distribution shift and applications to causal inference. This project will establish statistical theory for such methods, elucidating the respective roles of statistical invariance and sufficiency in modern machine learning, including approximate versions learned from data.
In addition to the theoretical objectives of the research project, Alexander will collaborate on work to develop theoretically sound representation learning methods for data compression and data-driven algorithm design. Such methods are at the forefront of research into data-driven solutions to core computer science problems, and pose challenging methodological and theoretical questions. Successful development of new methods will require designing appropriate deep neural network models and optimization objectives, implementing them to be trained efficiently on massive data sets, and running reproducible large-scale computer experiments to assess empirical performance. Moreover, collaborating on the release of clean, open-source code will be part of the project.
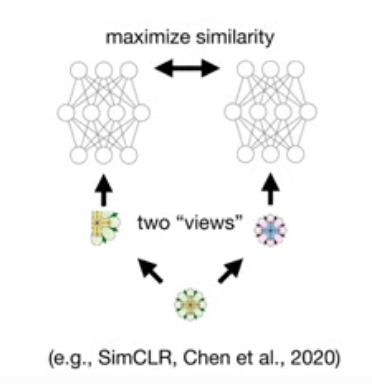
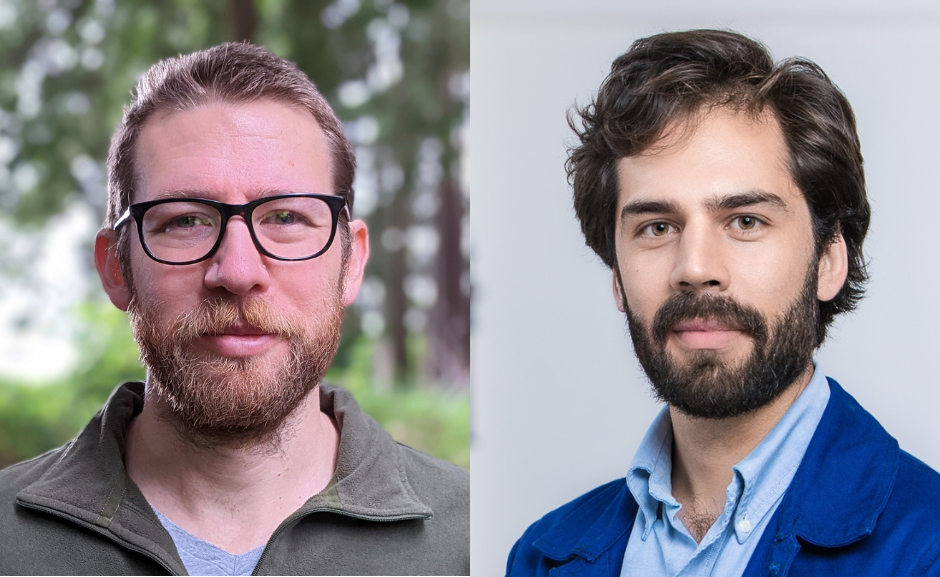
Getting to Know Alex
Alex Sharp’s first research experience came at St. Thomas University, where he participated in a project to model the profitability of a set of natural gas royalty regimes to be considered by the province of New Brunswick. This experience was “a great introduction for me to the utility of mathematics and statistics in informing real-world decision-making.”
Later he completed his master’s and PhD degrees at the University of Waterloo under the supervision of Dr. Ryan Browne. His research during this time focused mostly on theorizing and implementing parametric functional mixture models and led to two publications. He also had the chance to take a leadership role in collaborations with junior graduate students, leading to an additional publication with another soon to be submitted.
Alexander notes that his interest in the CDPF program comes from his desire to complement the “individualistic” research skills he has developed during his doctoral program with an experience that emphasizes research as a “collaborative enterprise, where many specialists contribute their expertise toward the advancement of a common goal.”
As a recipient of the CANSSI Distinguished Postdoctoral Fellowship, Alex will work under the supervision of Benjamin Bloem-Reddy at the University of British Columbia and Chris. J. Maddison at the University of Toronto
I am eager to apply the research skills I have honed to a problem that truly requires a collaborative and interdisciplinary approach—a problem that cannot be solved solely by my individual expertise.
About the Supervisors
Benjamin Bloem-Reddy
Benjamin Bloem-Reddy is an Assistant Professor in the Department of Statistics and an Associate Member of the Department of Computer Science at the University of British Columbia (UBC).
He works on problems in statistics and machine learning, with an emphasis on probabilistic approaches. Most of his ongoing (and future) work is motivated by some form of (scientific) knowledge acquisition, and the interrelated roles of models, data, inference, and prediction. Some recent examples:
- Uses and benefits of symmetry in modelling, inference, and computation
- Integrating probability, statistics, and data with scientific models
- The role of causality, broadly construed, in learning from observations of and interactions with the world
He also collaborates with researchers in the sciences on statistical problems arising in their research. His research is supported by funding from NSERC and UBC.
Chris J. Maddison
Chris Maddison is an Assistant Professor in the Department of Computer Science and the Department of Statistical Sciences at the University of Toronto. He is a CIFAR AI Chair at the Vector Institute, a research scientist at DeepMind, a member of the ELLIS Society, and a faculty affiliate of the Schwartz Reisman Institute for Technology and Society.
Chris works on the methodology of statistical machine learning, with an emphasis on methods that work at scale in deep learning applications. His research interests lie in the study of Bayesian inference, optimization, and discrete search.
Previously, he was a member at the Institute for Advanced Study in Princeton, New Jersey, from 2019–2020, and he completed his DPhil at the University of Oxford. He was an Open Philanthropy AI Fellow during his graduate studies. He received a NeurIPS Best Paper Award in 2014. He was a founding member of the AlphaGo project, which received the IJCAI Marvin Minsky Medal for Outstanding Achievements in AI in 2018.
Explore More Stories
Find Related Programs
“Estimating COVID-19 Prevalence in Homeless Populations” is a CANSSI Distinguished Postdoctoral Fellowship (CDPF) project. The CDPF is a two-year program that includes a substantial research project, applied interdisciplinary collaboration, and teaching experience.
CANSSI Distinguished Postdoctoral Fellowships are supported by a competitive salary. They provide opportunities for professional development and prepare postdoctoral fellows for success in a variety of careers.