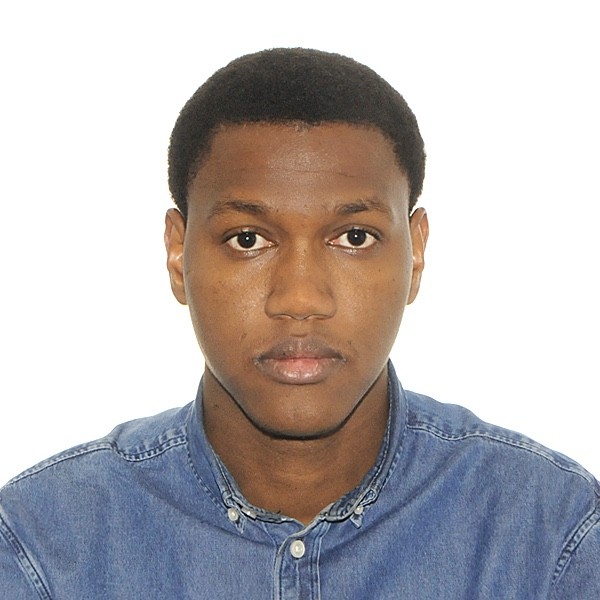
Post-Graduate Stories
Ismaïla Ba Explores “Zero-Inflation in Multinomial Principal Component Analysis for Microbiome Data”
In this project, Ismaïla will develop novel statistical methodology related to zero-inflated multinomial principal component analysis and will explore options for fitting the model through variational Bayes methods.
Program: CANSSI Distinguished Postdoctoral Fellowship
Region: National
Date: 2022–2024
Project Focus Areas
Dimension reduction techniques are among the most essential analytical tools in the statistical analysis of genomic data. Generalized principal component analysis is an extension to standard principal component analysis (PCA) for non-Gaussian data and has been used in the analysis of data from genomic platforms such as single-cell sequencing and microbial/metagenomic sequencing. In particular, multinomial PCA has been adapted for use in these contexts. In microbiome data, however, there is often an abundance of zero counts, which is not accounted for in the multinomial PCA framework. In this project, Ismaïla will develop novel statistical methodology related to zero-inflated multinomial PCA and will explore options for fitting the model through variational Bayes methods.
A common element of data arising from next-generation sequencing technologies is the presence of unmeasured factors affecting the observed data distributions. Such factors can be detected in an unsupervised manner using generalized Principal Component Analysis (gPCA). Ismaïla will perform methodological work to integrate the zero-inflation framework from previous research into the multinomial gPCA model. Specifically, he will first extend the gPCA framework to a zero-inflated generalized Dirichlet multinomial model.
However, the large number of parameters that need to be estimated in gPCA can lead to computational challenges. For this reason, Ismaïla will study and implement a variational EM algorithm for estimating the parameters of the gPCA model. A successful implementation of a variational EM algorithm will make this model appropriate for large-scale genomic studies.
The impact of this project will be a new technique for performing zero-inflated multinomial PCA in microbiome sequencing data. An R package will be designed for use by researchers in the biomedical sciences with a limited quantitative background. To the applicants’ knowledge, there is no such tool implementing this specific model available for biomedical researchers.
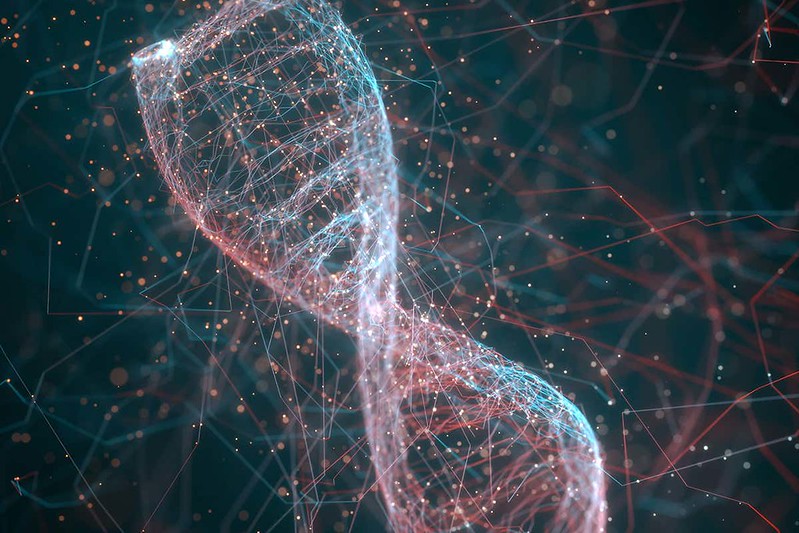
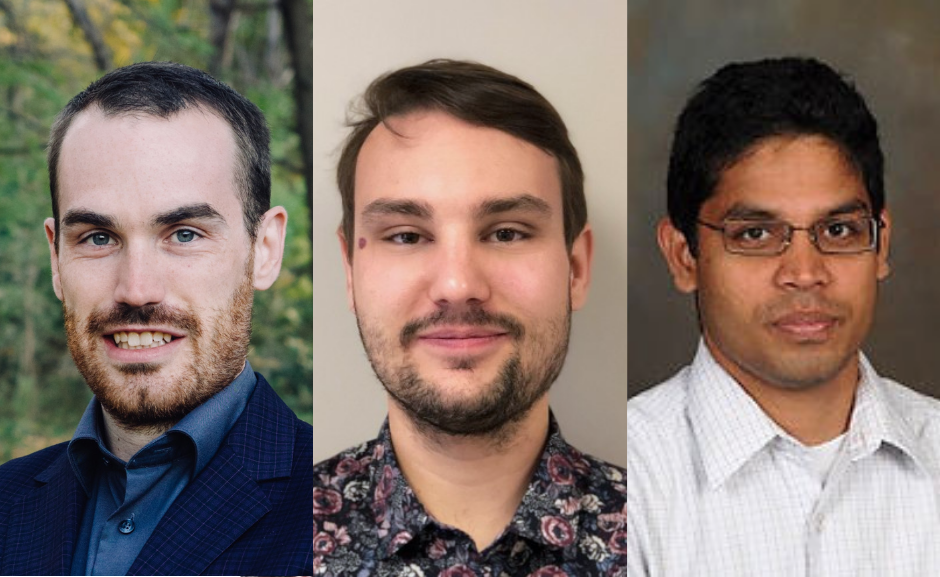
Getting to Know Ismaïla
Ismaïla Ba received his PhD in statistics from the Université du Québec à Montréal in 2022.
His long-term research interests include high-dimensional regression, feature screening and selection procedure, and development of cutting-edge machine learning methods with the goal of developing skills and knowledge for a career in the development of statistical methods and computational software for the analysis of high-dimensional data arising from a number of domains.
Ismaïla has a personal interest in science communication and would like to continue to take advantage of opportunities to exercise these skills by penning articles for blogs and online magazine and by presenting his research to non-expert audiences during his postdoctoral term.
As a recipient of the CANSSI Distinguished Postdoctoral Fellowship, Ismaïla will work under the supervision of Professor Kevin McGregor at York University and Professor Maxime Turgeon at the University of Manitoba.
About the Supervisors
Kevin McGregor
Kevin McGregor is an assistant professor in the Department of Mathematics and Statistics at York University in Toronto.
His research interests include:
- Microbiome data analysis
- Statistical genetics
- Analysis of high-dimensional data
- Network analysis in microbiome data
- Bayesian statistical methods
- Statistical analysis of DNA methylation data
Maxime Turgeon
Maxime Turgeon is an assistant professor in the Department of Statistics and the Department of Computer Science at the University of Manitoba in Winnipeg.
He received his PhD in Biostatistics from McGill University in 2019 while working under the supervision of Dr. Celia Greenwood and Dr. Aurélie Labbe. The title of his PhD thesis is Dimension Reduction and High-Dimensional Data: Estimation and Inference with Application to Genomics and Neuroimaging.
His main research interests are dimension reduction methods for high-dimensional data. This includes linear approaches (e.g., PCA, CCA, PCEV, PLS) as well as nonlinear approaches (e.g., manifold learning, autoencoders). High-dimensional data is challenging to analyze because of the so-called “curse of dimensionality.” However, this curse can be mitigated by using the structure in the data to develop new methods. Maxime is interested in developing statistical methodologies that are statistically and computationally efficient. He is also interested in applications to statistical genetics, genomics, neuroimaging, anomaly detection, and text data analysis.
Saman Muthukumarana
Saman Muthukumarana joined the Department of Statistics, University of Manitoba, as an assistant professor in July 2010. He was promoted to associate professor (with tenure) in 2016 and then to full professor in 2022.
Prior to that, he received a BSc honours special degree in Statistics from the University of Sri Jayewardenepura, Sri Lanka. He then completed an MSc (statistics) at Simon Fraser University in April 2007.
For his doctoral thesis, he continued to work with Dr Tim Swartz on Bayesian methods and applications and completed his PhD in June 2010.
Saman’s primary research interests lie broadly in Bayesian methods and computation for complex models which integrate multidisciplinary applications. Along with this main theme, he has developed methods to facilitate modelling and inference on non-standard complex data which lead to innovative analyses in the areas of social networks, health studies, sports, customer and user behaviour analytics, and environmental and ecological studies.
Explore More Stories
Find Related Programs
“Zero-Inflation in Multinomial Principal Component Analysis for Microbiome Data” is a CANSSI Distinguished Postdoctoral Fellowship (CDPF) project. The CDPF is a two-year program that includes a substantial research project, applied interdisciplinary collaboration, and teaching experience.
CANSSI Distinguished Postdoctoral Fellowships are supported by a competitive salary. They provide opportunities for professional development and prepare postdoctoral fellows for success in a variety of careers.