CANSSI’s Collaborative Research Teams (CRT) program supports sustained interdisciplinary collaborations that use statistical sciences to solve complex problems of broad importance for science, engineering, health, and society.
For the three-year period starting in 2025, CANSSI will fund three new CRT projects led by statisticians. They involve researchers from seven universities across Canada and promise to make important contributions in areas as diverse as agriculture, health care, and Bayesian inference. Here’s a quick overview of the projects and the project teams.
CRT 27: Fast, Distributed Bayes for Everyone
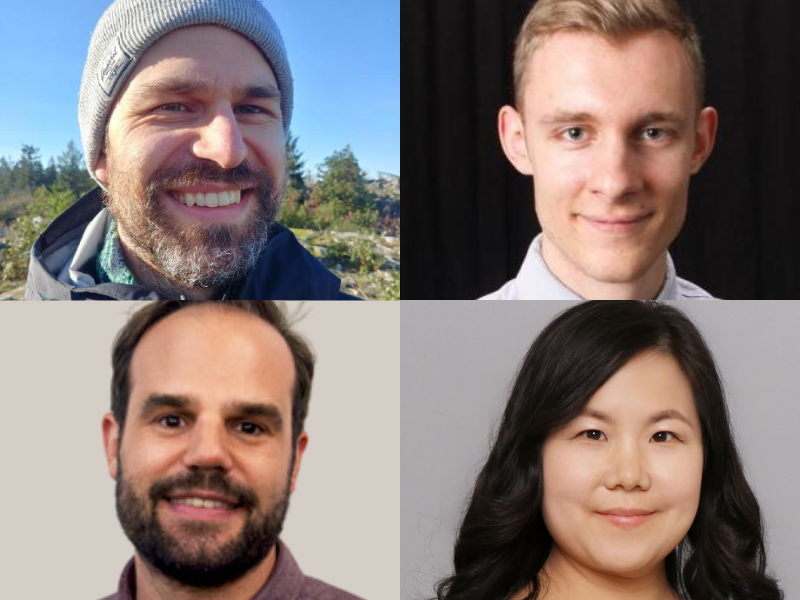
A team led by (clockwise from top left) Alexandre Bouchard-Côté (University of British Columbia), Trevor Campbell (UBC), Liangliang Wang (Simon Fraser University), and Philippe Gagnon (Université de Montréal) will explore ways to extend the use of computational Bayesian methods by leveraging modern, massively parallel distributed and cloud computing platforms. Their goal is to enable users to capitalize on the power of large-scale distributed computing platforms for Bayesian inference involving complicated posterior distribution, without needing any expertise in distributed computing.
CRT 28: Managing Data Complexities in Electronic Health Records: Statistical Strategies for Enhanced Learning
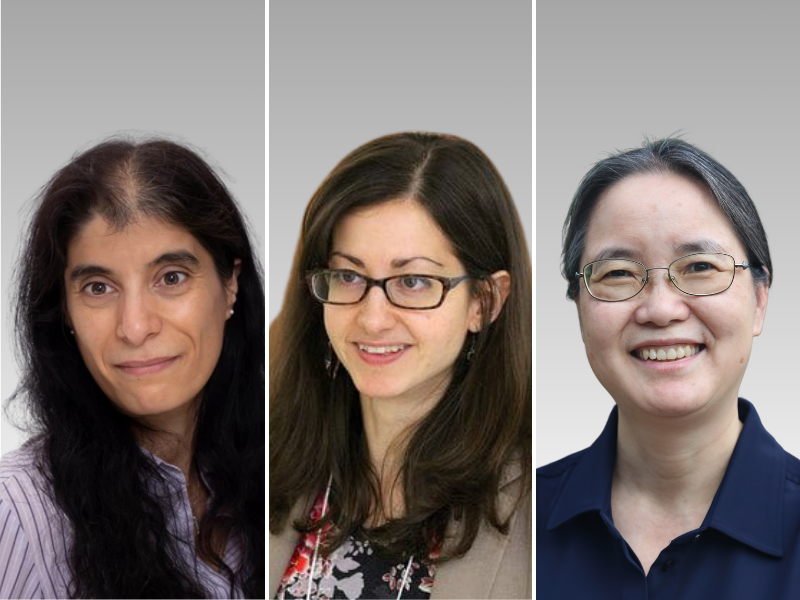
A team led by (left to right) Eleanor Pullenayegum (University of Toronto, The Hospital for Sick Children), Mireille Schnitzer (Université de Montréal), and Grace Y. Yi (University of Western Ontario) will develop new methods to manage the data complexities of electronic health records (EHR). In particular, they will address four problems encountered in EHR-based research led by their clinical collaborators:
- Causal inference with high-dimensional confounders
- Informative presence bias
- Handling visiting and missingness not at random
- Causal inference with time-varying longitudinal exposures
CRT 29: Statistical Methodologies and Computational Tools to Identify Microbial Correlates of Canadian Bee Gut Health
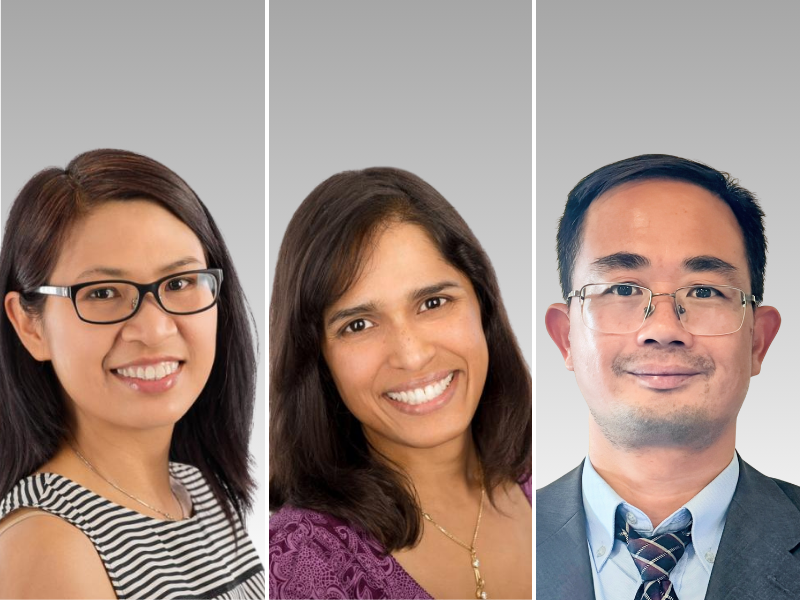
A team led by (left to right) Zeny Feng (University of Guelph), Ayesha Ali (Guelph), and Longhai Li (University of Saskatchewan) will build on work done by the Canadian Bee Gut Project to develop new methods for understanding the correlations between the composition of the bee gut microbiome and a set of covariates. More generally, the project will aim to “further advance the field of compositional data analysis by developing novel and more feasible statistical and computational methods to analyze complex multivariate count models.”