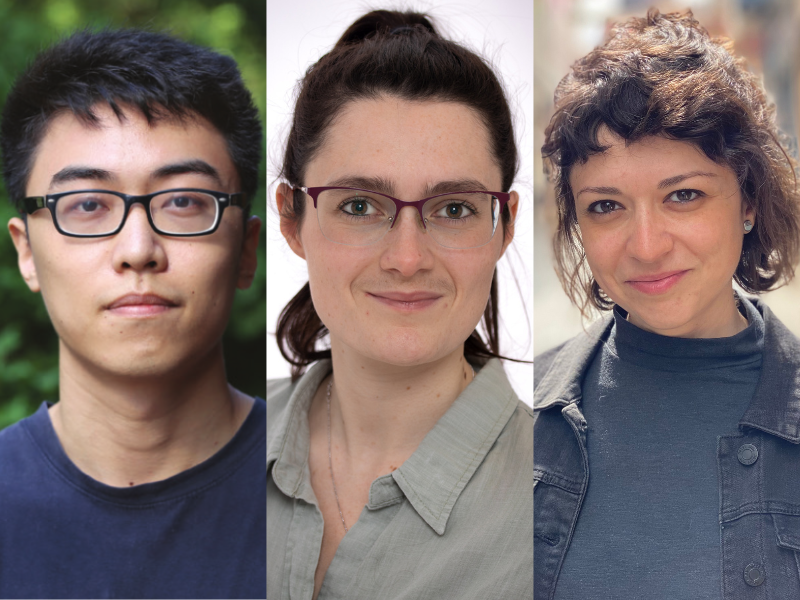
Collaborative Research Team Projects – Project 24
Statistical Design of Bayesian Adaptive Clinical Trials
This project will develop novel statistical methodology to improve the design of platform trials that minimize patient burden by efficiently answering multiple research questions, and dose-finding studies, to ensure safe and effective treatments are evaluated in later trials. We will also develop methodology to address the computational barriers to Bayesian adaptive trial design from two perspectives.
Research Category:
Region: National
Date: 2023–2026
Why Develop New Bayesian Adaptive Approaches for Clinical Trials?
Adaptive trials, such as those used during the development of COVID-19 vaccines, respond to accumulating data during the trial to efficiently reach conclusions, while maintaining valid statistical inference, and they make better use of time and money than fixed designs. The Bayesian approach is advantageous for adaptive trials as (i) parameter information can be continuously updated based on accumulating data, (ii) information can be synthesized across multiple sources, (iii) statistical efficiency can be improved using hierarchical modeling, and (iv) probabilistic statements about the effect of interest are more easily interpreted by clinicians
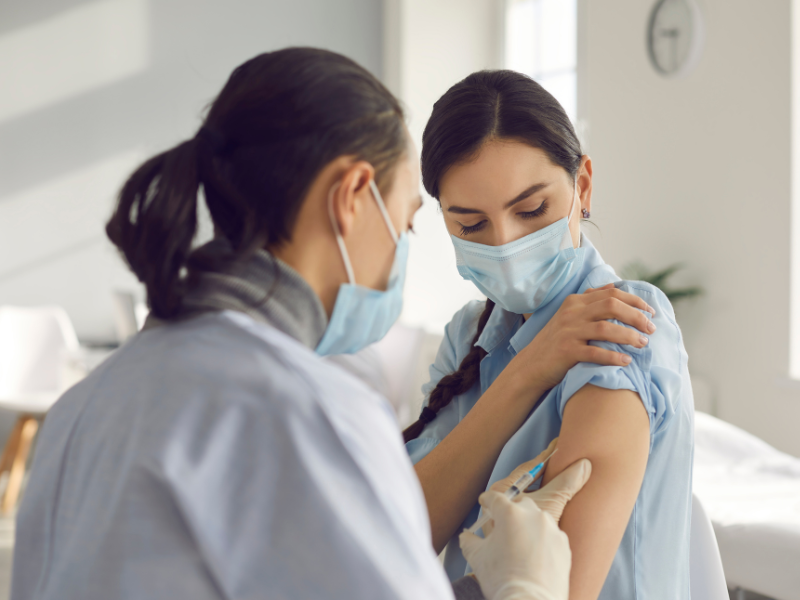
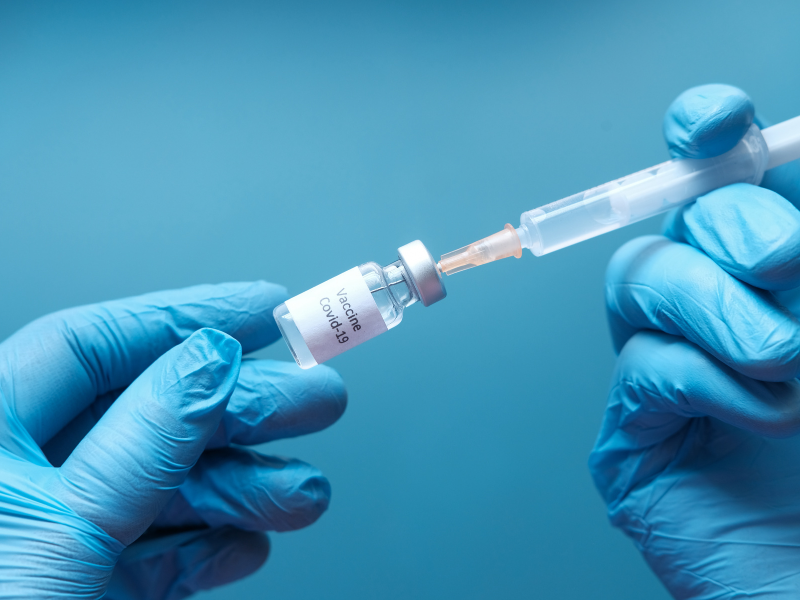
Areas of Exploration
Platform Trials
Platform trials aim to assess multiple research questions using a single trial infrastructure, but novel methodology is required to efficiently exploit this infrastructure and answer the proposed research questions with minimal patient burden and costs.
Dose Finding Trials
Statistical methods in dose finding trials aim to accurately determine the optimal dose based on a small number of patients. The proposed methodology will improve the chances of evaluating safe and effective treatments in later trials.
Computationally Efficient Design of Trials
Intensive simulations are currently required to design Bayesian adaptive trials. We aim to build on efficient Bayesian simulation algorithms to reduce computational complexity of these simulations and provide accurate uncertainty quantification to dramatically improve the feasibility of designing Bayesian adaptive trials.
Decision Theoretic Trials
Bayesian decision theory provides a principled framework for trial design, but its practical implementation is prevented by computational issues. Leveraging our work on efficient design of trials, we aim to develop novel algorithms to efficiently estimate key quantities and optimize Bayesian adaptive trials that support clinical decision making.
People Behind the Project
Project Team
Anna Heath | University of Toronto and The Hospital for Sick Children, Toronto
Shirin Golchi | McGill University
Haolun Shi | Simon Fraser University
Collaborators
George Tomlinson | University of Toronto (mentor)
Ying Yuan | University of Texas MD Anderson Cancer Center (mentor)
Terry Klassen | University of Manitoba
Rhonda Rosychuk | University of Alberta
Jean-Claude Tardif | University of Montreal
Corinne Hohl | University of British Columbia
Srinivas Murthy | University of British Columbia
Hawre Jalal | University of Ottawa (project information)
Ewan Goligher | University of Toronto (project information)
Jay Park | McMaster University (project information)