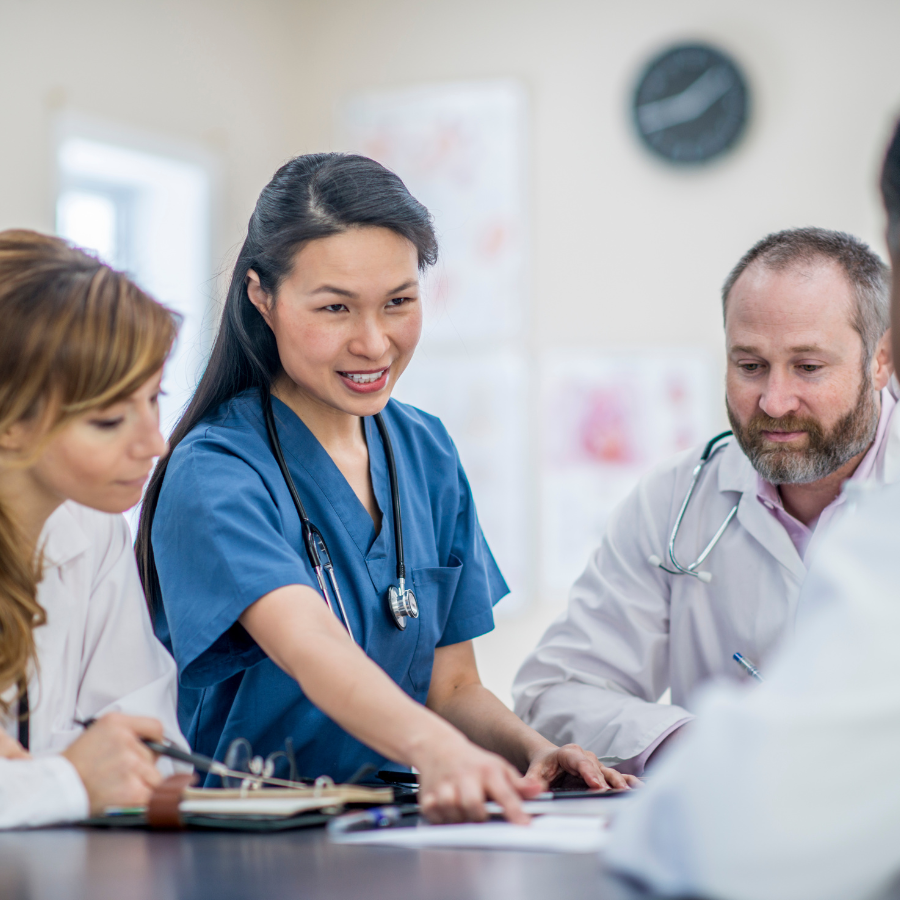
CANSSI Research for Social Good Program
Research for Social Good
Support for researchers who are supporting society.
This program supports researchers in statistical sciences poised to have an immediate impact on problems that are important to society. It focuses on research that is strongly engaged with collaborators engaged in social issues.
- Support Amount: Depends on scope of project and number of students and postdoctoral fellows involved
- Region: National
- Deadlines: Applications are reviewed on a rolling basis
- Program Duration: 2–12 months
- Eligibility: Faculty, students and postdoctoral fellows
Have questions about the program?
Overview
The Research for Social Good Program is designed to support statistical scientists at CANSSI member institutes who are in a position to provide critical research support on emerging problems with immediate impact on issues important to society.
The emphasis is on supporting collaborative research that is important to society on a provincial, national, and/or international scale, especially projects focused on social issues
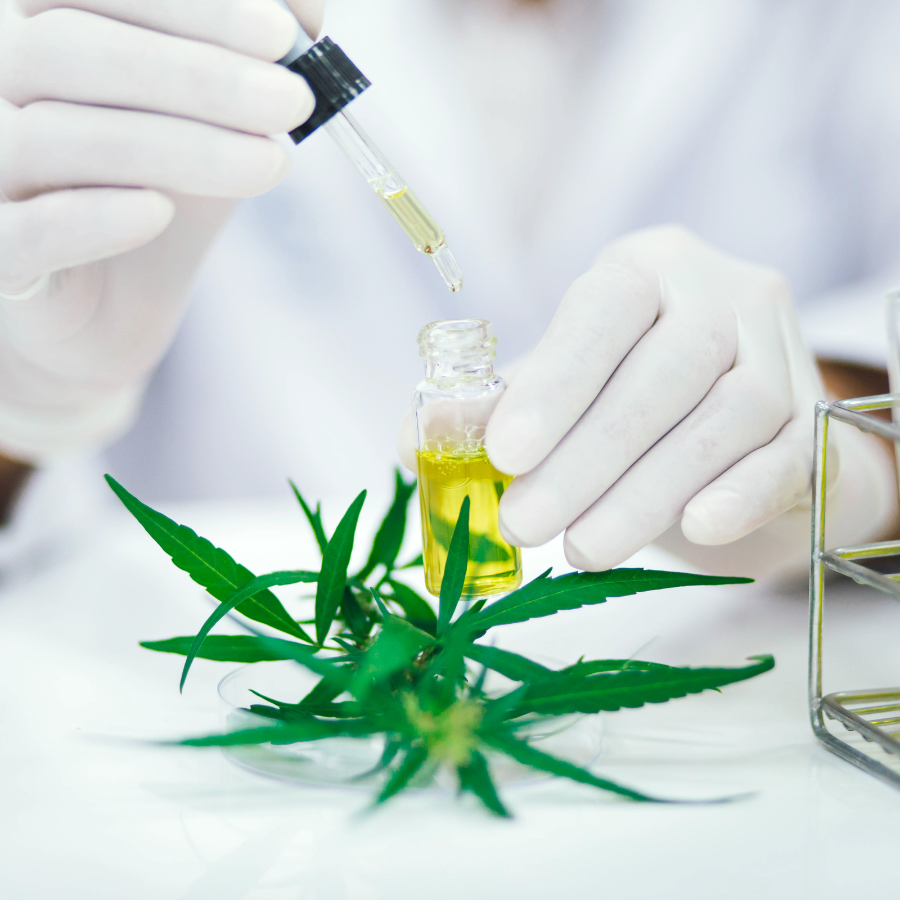
What’s Involved
Identifying Suitable Problems
Faculty interested in obtaining support under the Research for Social Good Program should contact the Directors to discuss the potential project.
Applying to the Program
An application consists of a 1-2 page description of the research, the potential for immediate impact, and the equity, diversity, and inclusion (EDI) aspects of the proposed project, the CANSSI biographical form for the statistical sciences leads, a description of the application partners and their qualifications for the proposed project, and a short budget indicating specific students and postdoctoral fellows to be supported. CANSSI Directors are happy to advise leads preparing an application.
Applications to the Research for Social Good Program are evaluated by the CANSSI Directorate.
Reporting
Supported investigators will write a short report describing:
- The results
- The extent to which the goal of having an immediate impact was achieved
- The potential for future projects
Deadline for Reporting: Report expected four weeks after the project ends
Who’s Eligible
Faculty Members
Lead investigators must be statistical scientists at CANSSI member institutes. They must be in the position to provide critical research support on rapidly emerging problems important to society, focusing on applied research that has strong potential to have an immediate impact.
Proposals are submitted by faculty members.
Students and Postdoctoral Fellows
Supported investigators may be students and/or postdoctoral fellows. These participants are selected by the lead investigator(s) and are typically noted in the application.
Equity, Diversity, and Inclusion (EDI)
The Research for Social Good Program is an important part of CANSSI EDI. The impact of supported projects usually has direct and/or indirect consequences for diversity and equity issues. Project participants take part in the CANSSI EDI program as specified in the program requirements.
How to Apply
Please contact the Director to discuss potential projects.
Budget
Project budgets depend on the scope of the problem and the number of students and postdocs that will be supported. Projects may last from 2 to 12 months. Projects are non-renewable.
This budget can be used to support graduate students and postdoctoral fellows, as well as the costs of data acquisition over the course of the project.
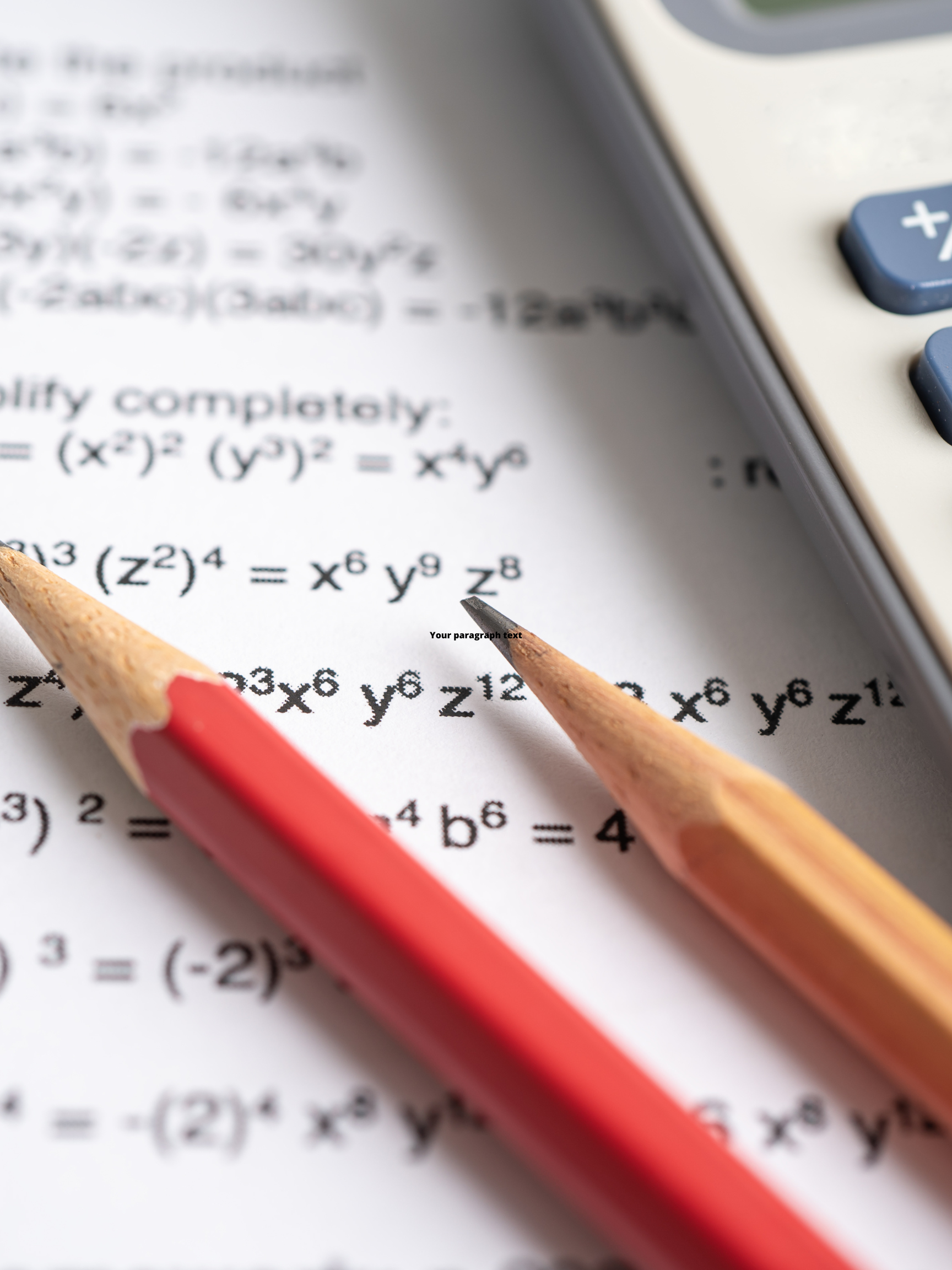
Review
Applications are reviewed by the CANSSI Directorate.
Past RSG Projects
Estimating the Number of Hidden COVID-19 Cases
Many cases of COVID-19 have gone undetected. This is due to factors such as the volume of virus testing, asymptomatic patients, incorrect self-diagnosis, or failure to disclose infection to a health authority. Undetected cases may drive community infections and reduce the effectiveness of control measures. In order to investigate the rates of under-reporting of COVID-19 in Canada, CANSSI members will be adapting their methods from wildlife ecology to better quantify the real scope of this pandemic. This project is led by Laura Cowen, Junling Ma and Pauline van den Driessche, who are all from the University of Victoria.
Currently, only people with symptoms are being tested for COVID-19, which likely means there are hidden cases in Canada due to the current testing approach. By adapting hidden-population methods, which are used in studies that involve injection drug users and the homeless population, this team aims to uncover more data about hidden COVID-19 cases. This will enable health authorities to make more informed public health policies in the current and future waves of COVID-19. Understanding the scope of this disease is vital in assessing current health care and social responses. This work can also be used to better understand under-reporting of future pandemics.
Host Genetics for SARS-CoV-2 Severity and Infection: Power and Control
People who have contracted COVID-19 may have important information about their genetic makeup that gives researchers more insight into how the disease develops in an individual’s body. Led by Lloyd T. Elliot from Simon Fraser University, this team aims to better understand how people’s different genetic variations can inform COVID-19 vaccine and drug development. Specifically, Lloyd and his team will perform preliminary studies on genes involved in the infectious pathways of coronaviruses such as angiotensin-converting enzyme 2.
In addition, this team is using data from the UK Biobank to better understand and share how factors like ethnicity and socioeconomic status can impact research that investigates human genetics’ role in the body’s response to COVID-19. Because this pandemic is only just leaving its early stages, infections follow geographic and family lines. This means researchers need to control for socioeconomic structures to ensure that the solutions developed to address COVID-19 are accurate and consider a diverse population.
Improved Estimation of Social Contact Matrices for Modeling the COVID-19 Epidemic in Canada
Physical distancing is important in order to slow down and control the spread of COVID-19. Without a widely available vaccine, reducing physical contact is crucial, hence the importance of estimating the number of social contacts by population surveys. This project will aim to publish social contact matrices that will be shared with mathematical modellers and Canadian public health authorities to model the evolution of the COVID-19 pandemic.
The social contact matrices will use CONNECT survey data before and during confinement in Canada to establish expected contacts with re-openings currently underway across Canada. This data will then be integrated into this team’s dynamic mathematical model of transmission of COVID-19, developed specifically to guide decision-making in public health in Quebec. Students in this project are led by Alexandre Bureau, Marc Brisson and Mélanie Drolet of the Université Laval. This project, working in collaboration with the Institut national de santé publique du Québec, will enable health authorities to make more data-informed decisions in their physical-distancing measures and strategies as well as the gradual lifting of these measures.
Statistical Methods for COVID-19 Mortality Forecasting at the Small-Area Level
The Centre for Global Health Research has recently developed the Global COVID-19 Assessment of Mortality (GCAM) tool, which is an open, transparent, and continuously updated statistical model that combines actual COVID-19 mortality counts with Bayesian inference to forecast COVID-19 deaths.
Led by Jeffrey Rosenthal and Patrick Brown from the University of Toronto, students will improve the current GCAM tool by creating spatio-temporal predictions of COVID-19 mortality at the small area level with a hierarchical model; and accounting for irregularities in reporting times of non-hospital mortality by treating true event dates as latent variables. By improving this tool, this will allow the team to make the best possible use of COVID-19 mortality data as the pandemic spreads through its first wave, aiding countries in understanding national disease trajectories, and setting the stage for anticipating the course of future waves.
Investigating Exposure and Host Genetic Risk Factors in Studies of COVID-19
In the face of the COVID-19 pandemic, researchers have identified various characteristics that may influence the risk and potential for exposure for an individual to contract the virus. These covariates are essential in determining the presence of underlying conditions in addition to COVID-19, as well as for improving disease prediction. This research led by Lei Sun and Lisa Strug will provide this data to be incorporated into the Canadian COVID-19 Host Genome Sequencing Genome-Wide Association Study effort.
Secondly, these researchers are performing a study that will specifically identify genetic risk factors for COVID-19, including an analysis of sex-chromosomes, which can often be neglected from scientific studies. The impact of this project will be a robust analytical source to help increase accurate predictions of COVID-19 risk based on these risk factors.
New 2020 Mortality Tables for Quebec: Excess Mortality Due to COVID-19 and its Indirect Effects
Out of all the provinces in Canada, Quebec has been the hardest-hit by the COVID-19 pandemic. As of September 2020, Quebec accounted for the overwhelming majority of COVID-19-related deaths, prompting the need for updated life tables in light of the province’s death rates which have been affected by the virus. As a vaccine for COVID-19 is not expected until 2021 at the earliest, death rates will continue to be affected by the virus for the foreseeable future until the entire population is immunized.
Students in this research project will construct updated life tables for the province of Quebec, using information that takes into account the increased mortality due to COVID-19 as well as an individual’s age and sex. Statistical information concerning death rates is integral to actuarial work. This includes life insurance companies who use this data to calculate life insurance premiums, as well as The Canadian Institute of Actuaries and the Society of Actuaries which will use the methodology developed amongst other geographic regions. The project is led by Louis Doray, Jean-François Angers and Nadine Ouellette.
Can the Reported COVID-19 Data Tell us the Truth?
The effective management of the COVID-19 pandemic relies on accurate reporting of COVID-19 data. However, the collected data generally contains errors, and tends to under-report case numbers due to a current inability to address the number of asymptomatic infected cases. If the analyzed data remains unreliable, it becomes impossible to determine judgement for timely and effective infection control.
In order to address this problem, Grace Yi and Wenqing He are working with graduate students to analyse COVID-19 data using measurement error models to eliminate discrepancies and highlight faults in the data. This will provide a clearer understanding of the case fatality rate, which is the ratio of the number of deaths to the number of infected cases. Strengthening the quality of the data that informs our knowledge of the virus will lead to tangible insights that aid health care professionals in the COVID-19 response effort.
Modeling and Visualization of the COVID-19 Outbreak in Canadian Long-Term Care Homes
COVID-19 has created a tragic impact in long-term care homes in Canada, accounting for an estimated 50 percent of COVID-19-related deaths. In order to address this problem, factors associated with the potential for a home to have an outbreak must be assessed. Using publicly available data from Statistics Canada and the Government of Ontario, this project will analyze geographical, operational, and structural factors that contribute to the spread of COVID-19 in long-term care homes, in order to better understand how databases can be put to use in combating the spread in a meaningful way.
John Braun, Patrick Brown and Ngan Lyle will work with graduate students to assemble a database for the province of British Columbia to aid in the expansion of data analytics regarding COVID-19. This research will play a significant role in guiding intervention tactics that will reduce the impact of COVID-19 over the long-term presence of the virus.
Detecting Anomalies in COVID-19 Indicators
Building on a COVID-19 modeling project being undertaken by the DELPHI epidemiological forecasting team at Carnegie Mellon University, Daniel McDonald and his team will develop and implement statistical procedures that flag potential data quality issues, before it is used by DELPHI or released to the public through the API. A challenge the modelling team at DELPHI faces is the rapid identification of anomalous indicator values, which can cause data quality issues for internal and external data consumers since this data is made public without any lag. This makes it difficult to systematically and rapidly catch these issues.
The results of this project will be used internally by the modelling team and integrated into the forecast pipeline. Additionally, Daniel’s team will work with the visualization team to incorporate the developed diagnostic tools into the Covidcast site for the benefit of external data consumers.